Combining domain filling with a self-organizing map to analyze multi-species hydrocarbon signatures on a regional scale
For the period of the Barnett Coordinated Campaign, October 16–31, 2013, hourly concentrations for 46 volatile organic compounds (VOCs) were recorded at 14 air monitoring stations within the Barnett Shale of North Texas. These measurements are used to identify and analyze multi-species hydrocarbon signatures on a regional scale through the novel combination of two techniques: domain filling with Lagrangian trajectories and the machine learning unsupervised classification algorithm called a self-organizing map (SOM). This combination of techniques is shown to accurately identify concentration enhancements in the lightest measured alkane species at and downwind of the locations of active-permit oil and gas facilities, despite the model having no a priori knowledge of these source locations. Site comparisons further identify the SOM’s ability to distinguish between signatures with differing influences from oil- and gas-related processes and from urban processes. A random forest (a machine learning supervised classification) analysis is conducted to further probe the sensitivities of the SOM classification in response to changes in any hydrocarbon species’ concentration values. The random forest analysis of four representative classes finds that the SOM classification is appropriately more sensitive to changes in certain urban-related species for urban-related classes, and to changes in oil- and gas-related species for oil- and gas-related classes.
Nathan, B.J., Lary, D.J. Combining domain filling with a self-organizing map to analyze multi-species hydrocarbon signatures on a regional scale. Environ Monit Assess 191, 337 (2019). https://doi.org/10.1007/s10661-019-7429-9
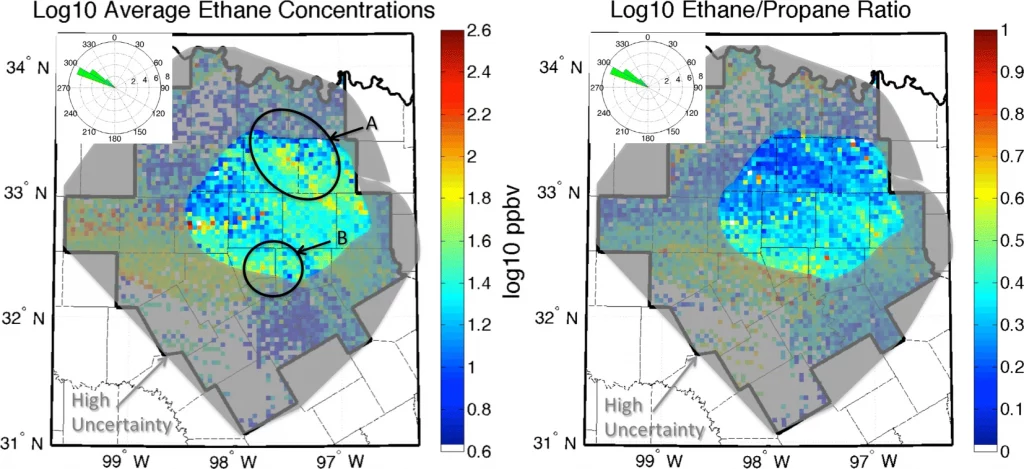